Excel at Monopoly #1: Who Wants to Play a Game?
17 August 2016
If you’re like most normal people, you probably would have seen the tag line and immediately run a million miles away from the site. But if you’re reading this, you’re clearly either an Excel geek, a Monopoly geek, or both.
Before we jump into the Excel side of things, let’s start off looking at Monopoly. Most people assume that Monopoly is a game of chance. That means that everybody has an equal chance to win, right? Just like Blackjack and Poker are games of chance, right?
Imperfect information
Your dice rolls are up to chance, just like the cards that you’re dealt at the casino. But the decisions you make will have more sway on the result of the game, just as they do in Blackjack and Poker. Sure, if by some miracle, you land on Community Chest, Income Tax and then head straight to Jail on the first round (about 1 in 7500 chance of that – you’d think you’d see it happening more often, actually), you’ll be in a weaker position. But you can always claw your way back with some good decision making and solid trades. Like any game of so-called ‘chance’, having information and using it well will always swing the game in your favour.
If you have good information, you have a better chance at winning. So, to excel at Monopoly, the trick is to understand what properties are good, in order to make better decisions when buying and trading.
How might we define a good property? It might be:
- A property that people land on frequently
- A property that charges high rental
- A property that isn’t too expensive to purchase
This week, we’ll just start looking at the first step. How can we identify which squares people land on more frequently? There are 40 squares on the board, each one should be equally likely, right?
That couldn’t be further from the truth.
Without knowing
If we look at the board as a whole, we can see that there are some squares with markedly lower probabilities – notably Chance squares and “Go to Jail” (it’s pretty hard to end your turn there!) – and one with a very high probability (who hasn’t spent turns sitting around in Jail?). Everything else somewhat blends in with each other, relative to the highs and lows of the other squares.
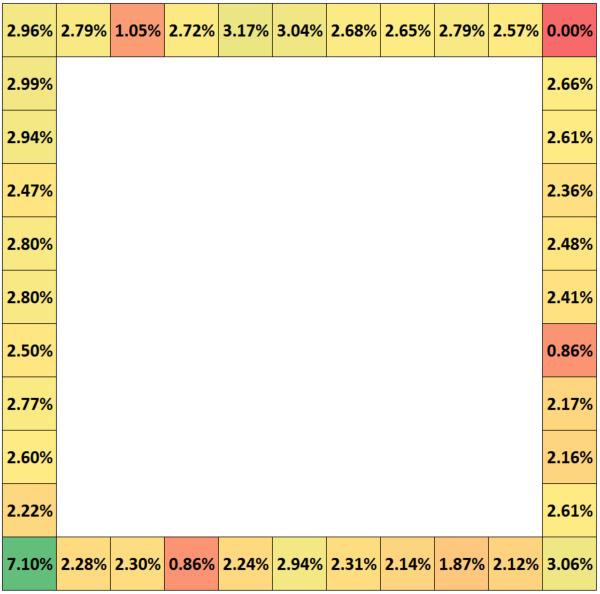
Now, if we remove the non-purchasable properties, you can see a much greater deviation between high likelihood squares and low likelihood squares. Properties along the bottom row, for example, are much less likely to be landed on, with the exception of King’s Cross Station or Reading Railroad (or other similar station, depending on your local version of the game). You’ve got a 50% higher chance of landing on Trafalgar Square than you do on Old Kent Road (or Illinois Avenue and Mediterranean Avenue, for our US friends).
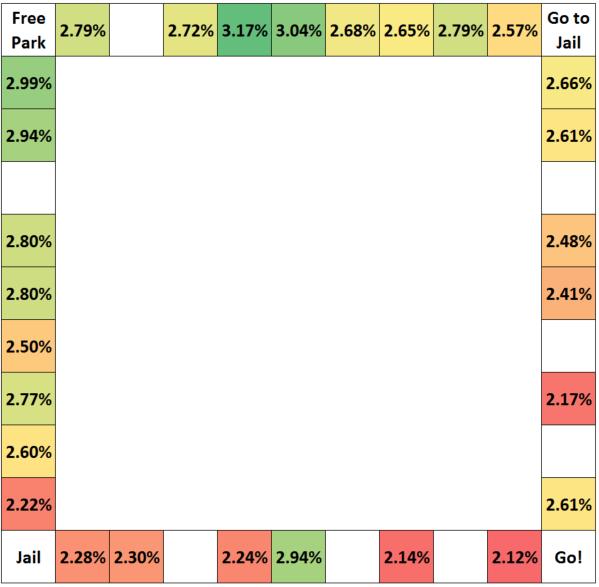
Where to from here?
So why is this the case? What causes these differences, and how can we work these out? And most importantly, how can understanding these help us in our game? Over the next 4 weeks, we’ll explain the answers to these questions, showing you how you can calculate your own probabilities (these actually change as the game rules change, version to version), and develop expected value and payoff periods for each of the properties.
I hope you’ll play along with us at home!